CRISP-ML(Q) - Business Understanding and Data Understanding
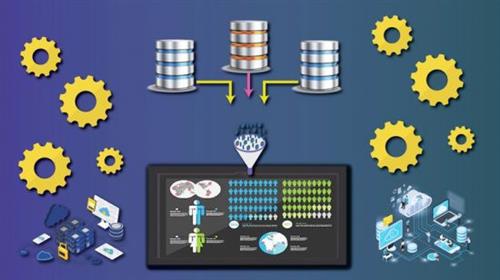
Free Download CRISP-ML(Q) - Business Understanding and Data Understanding
Last updated 6/2023
MP4 | Video: h264, 1280x720 | Audio: AAC, 44.1 KHz, 2 Ch
Language: English | Duration: 4h 3m | Size: 2.3 GB
Data Science - Business Understanding and Data Understanding
What you'll learn
Understanding project management methodology to handle data related projects.
Understand business problem definition.
Understand data types as well as data collection mechanisms.
Understand exploratory Data Analytics (EDA) / Descriptive statistics as well
Understand the various Data cleaning / Pre-processing tasks Using Python.
Requirements
No programming and no statistics knowledge required.
Everything will be taught here from the very begining.
Basic computer Knowledge and primary school Mathematics knowledge is sufficient.
Description
This course will help you understand the basics of Data Science and EDA using Python and we shall also dive deep into the Project Management Methodology, CRISP-ML(Q). Cross-Industry Standard Process for Machine Learning with Quality Assurance is abbreviated as CRISP-ML(Q). Data Science is omnipresent in every sector. The purpose of Data Science is to find trends and patterns with the data that is available through various techniques. Data Scientists are also responsible for drawing insights after analyzing data. Data Science is a multidisciplinary field that involves mathematics, statistics, computer science, Python, machine learning, etc. Data Scientists need to be adept in these topics. This course will provide you with an understanding of all the aforementioned topics.
A detailed explanation of the 6 stages of CRISP-ML(Q) will be provided. These 6 stages are as follows
Business and Data Understanding
Data Preparation
Model Building
Evaluation
Model Deployment
Monitoring & Maintenance
The importance of Business objectives and constraints, Business success criteria, Economic success criteria, and Project charter will be thoroughly understood. Elaborate descriptions of various data types - continuous, discrete, qualitative, quantitative, structured, semi-structured, unstructured, big, and non-big data, cross-sectional, time series and panel data, balanced and unbalanced data, and finally, offline and live streaming data. Various aspects of data collection will be looked into. Primary, and secondary, data version control, description, requirements, and verification will be analyzed.
Data Preparation involving data cleansing, EDA using Python or descriptive statistics, and feature engineering will be elaborately explained. Data cleansing involves numerous methods like typecasting, handling duplicates, outlier treatment, zero & near zero variance, missing values, discretization, dummy variables, transformation, standardization, and string manipulation. The realm of EDA using Python will be explored, This would include understanding measures of central tendency (mean, median, and mode), measures of dispersion (variance, standard deviation, and range), skewness, and kurtosis which are also termed first, second, third and fourth-moment business decisions. More about bar plots, Q-Q plots, box plots, histograms, scatter plots, etc., will be looked into in EDA using Python. Feature engineering, the last part of data cleansing, will also be given enough coverage.
Further, the model building also known as data mining or machine learning will also be thoroughly talked about. Model building involves supervised learning, unsupervised learning, and, forecasting which will be explored. Several model-building techniques like Simple Linear regression, Multilinear regression, Logistic regression, Decision-Tree, Naive Bayes, etc.
The last few steps of CRISP-ML(Q) are Evaluation, Model Deployment, and Monitoring & Maintenance.
The learning journey will include CRISP-ML(Q) using Python & Data Science and EDA using Python. Having a thorough understanding of these topics will enable you to build a career in the field of data science.
Who this course is for
Beginners, Intermediate as well as advanced leaners.
Freshers who are new of data science and want to embark into the field of data science.
Working professionals who are working in different industries.
Lectures, Professors & Teachers whose primary role is to teach students on data related concepts.
Homepage
https://www.udemy.com/course/crisp-mlq-business-understanding-and-data-understanding/
Rapidgator
fagri.CRISPMLQ..Business.Understanding.and.Data.Understanding.part3.rar.html
fagri.CRISPMLQ..Business.Understanding.and.Data.Understanding.part2.rar.html
fagri.CRISPMLQ..Business.Understanding.and.Data.Understanding.part1.rar.html
Uploadgig
fagri.CRISPMLQ..Business.Understanding.and.Data.Understanding.part1.rar
fagri.CRISPMLQ..Business.Understanding.and.Data.Understanding.part2.rar
fagri.CRISPMLQ..Business.Understanding.and.Data.Understanding.part3.rar
NitroFlare
fagri.CRISPMLQ..Business.Understanding.and.Data.Understanding.part3.rar
fagri.CRISPMLQ..Business.Understanding.and.Data.Understanding.part2.rar
fagri.CRISPMLQ..Business.Understanding.and.Data.Understanding.part1.rar
Links are Interchangeable - Single Extraction
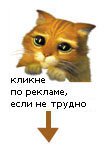