Causal Ai: An Introduction
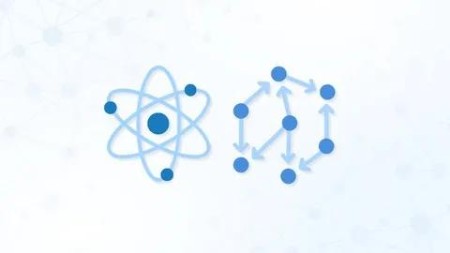
Causal Ai: An Introduction
Published 8/2024
MP4 | Video: h264, 1920x1080 | Audio: AAC, 44.1 KHz
Language: English | Size: 1.77 GB | Duration: 6h 45m
Learn the foundational components of Causal Artificial Intelligence
What you'll learn
What Causality is
The relationship between Causation and Association
Why RCT's are the golden standard for Causal Inference
Main components of Pearlian Framework for Causality: Ladder of Causation, Causal Graphs, Do-calculus, Structural Causal Models
Machine Learning & Propensity Score-based Causal Effect Estimators
Causal Discovery (Algorithms)
How to estimate Average Causal Effects using observational data (covering the entire end-to-end process)
Requirements
Basic Probability and Statistics knowledge
Description
In this course, you'll learn the foundational components of Causal Artificial Intelligence (Causal AI). More and more people are starting to realise that correlation-focused models are not enough to answer our most important business questions. Business decision-making is all about understanding the effect different decisions have on outcomes, and choosing the best option. We can't understand the effect decisions have on outcomes with just correlations; we must understand cause and effect. Unfortunately, there is a huge gap of knowledge in causal techniques among people working in the data & statistics industry. This means that causal problems are often approached with correlation-focused models, which results in sub-optimal or even poor solutions. In recent years, the field of Causality has evolved significantly, particularly due to the work of Judea Pearl. Judea Pearl has created a framework that provides clear and general methods we can use to understand causality and estimate causal effects using observational data. Combining his work with advances in AI has given rise to the field of Causal Artificial Intelligence.Causal AI is all about using AI models to estimate causal effects (using observational data). Generally, businesses rely only on experimentation methods like Randomized Controlled Trials (RCTs) and A/B tests to determine causal effects. Causal AI now adds to this by offering tools to estimate causal effects using observational data, which is more commonly available in business settings. This is particularly valuable when experimentation is not feasible or practical, making it a powerful tool for businesses looking to use their existing data for decision-making.This course is designed to bridge the knowledge gap in causal techniques for individuals interested in data and statistics. You will learn the foundational components of Causal AI, with a specific focus on the Pearlian Framework. Key concepts covered include The Ladder of Causation, Causal Graphs, Do-calculus, and Structural Causal Models. Additionally, the course will go into various estimation techniques, incorporating both machine learning and propensity score-based estimators. Last, you'll learn about methods we can use to obtain Causal Graphs, a process called Causal Discovery.By the end of this course, you'll be fully equipped with all tools needed to estimate average causal effects using observational data. We believe that everyone working in the data and statistics field should understand causality and be equipped with causal techniques. By educating yourself early in this area, you will set yourself apart from others in the field. If you have a basic understanding of probability and statistics and are interested in learning about Causal AI, this course is perfect for you!
Overview
Section 1: Causality, Association & RCT's
Lecture 1 Welcome
Lecture 2 Course Slides
Lecture 3 What is Causal AI?
Lecture 4 Simpson's Paradox
Lecture 5 The Need for Causality in Business
Lecture 6 Causation and its relation to Association
Lecture 7 RCT's: The Golden Standard for Causal Inference
Lecture 8 Course Outline
Section 2: The Ladder of Causation
Lecture 9 Introduction
Lecture 10 Layer 1 Explained
Lecture 11 Layer 1 Techniques
Lecture 12 Layer 2 Explained
Lecture 13 Layer 2 Techniques
Lecture 14 Layer 3 Explained
Lecture 15 Layer 3 Techniques
Lecture 16 Do-operator in light of Structural Causal Models
Lecture 17 Recap
Section 3: Causal Directed Acyclic Graphs
Lecture 18 Introduction
Lecture 19 What are Causal DAGs?
Lecture 20 Do-operator in light of Causal DAGs
Lecture 21 Graph Independence & Information Flows
Lecture 22 Graph Patterns
Lecture 23 Blocking Paths & D-separation
Lecture 24 From Graph (In)dependence to Statistical (In)dependence
Lecture 25 Recap
Section 4: Causal Inference Part 1: Identification
Lecture 26 Introduction
Lecture 27 Estimand & Conditional Ignorability
Lecture 28 Probabilities as the foundation of Causal Quantities
Lecture 29 Backdoor Adjustment
Lecture 30 Frontdoor Adjustment
Lecture 31 Do-calculus
Lecture 32 Positivity/Unconfoundedness Trade-Off
Lecture 33 Recap
Section 5: Causal Inference Part 2: Estimation
Lecture 34 Introduction
Lecture 35 Causal Quantities of Interest
Lecture 36 S-Learner
Lecture 37 T-Learner
Lecture 38 X-Learner
Lecture 39 Matching
Lecture 40 Inverse Probability Weighting
Lecture 41 Systematic vs. Random Errors
Lecture 42 Recap
Section 6: Causal Discovery
Lecture 43 Introduction
Lecture 44 Domain Expertise
Lecture 45 Causal Discovery Algorithms: Categories
Lecture 46 Causal Discovery Algorithms: Assumptions
Lecture 47 Constraint-based Causal Discovery
Lecture 48 Score-based Causal Discovery
Lecture 49 Function-based Causal Discovery
Lecture 50 Continuous Optimization-based Causal Discovery
Lecture 51 Causal Discovery in Practice: Hybrid & Iterative
Lecture 52 Recap
Section 7: Closure
Lecture 53 Introduction
Lecture 54 Challenges with Causal AI
Lecture 55 Considerations, Recommendations & Closure
Everyone interested in learning about Causal AI and who has some basic knowledge of Probability and Statistics,Particularly relevant for those working in the Data & Statistics field, like Data Scientists, Data Analysts, Decision Scientists, Statisticians, Data Engineers, Machine Learning Engineers, Computer Scientists, Business Intelligence Analysts, Quantitative Analysts, etc.,Those who want to be at the forefront of advancements in Data and AI for decision-making
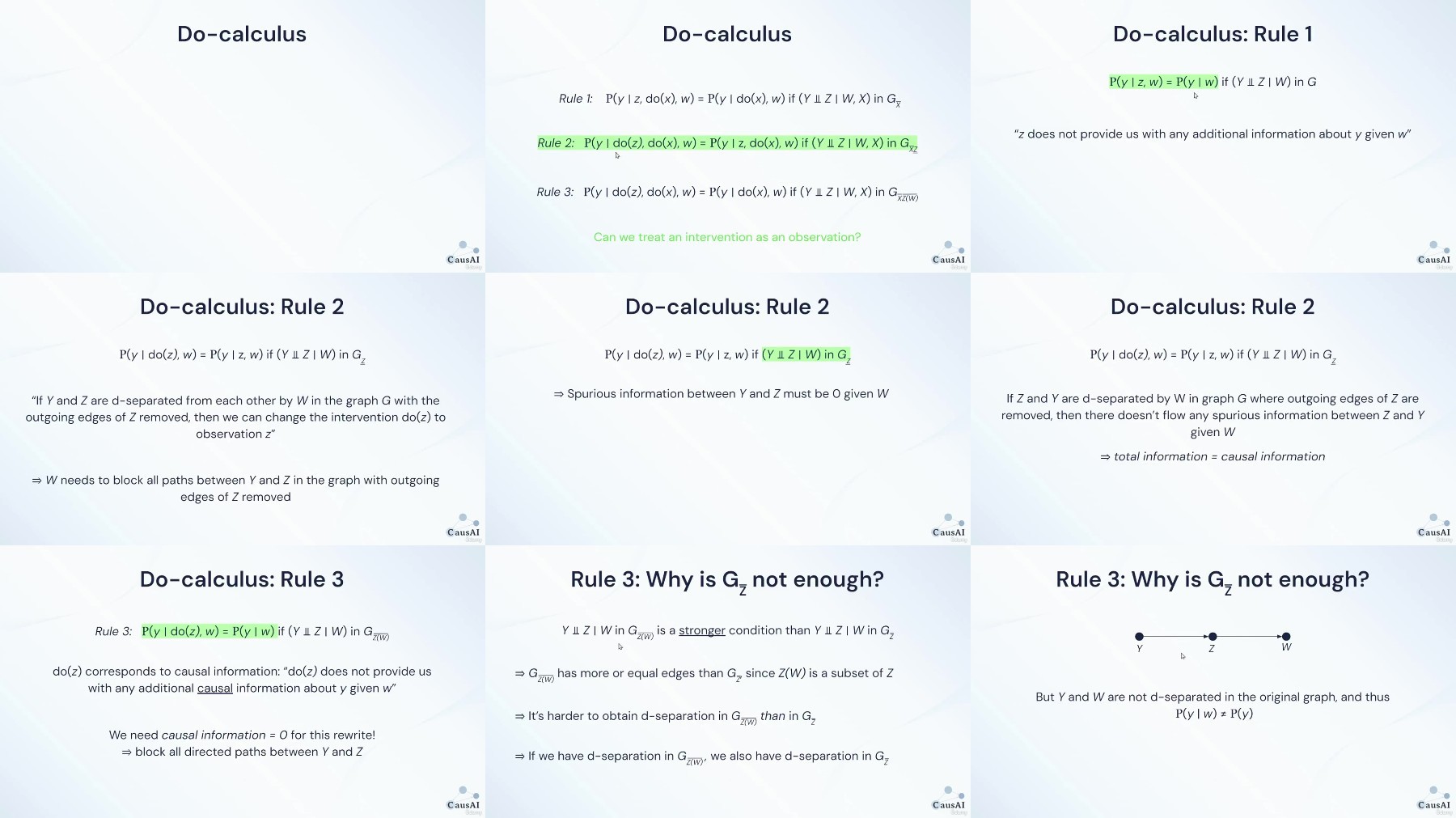
https://ddownload.com/mki84apsb1bo/Linkedin.-.Causal.AI.-.An.Introduction.part1.rar
https://ddownload.com/kulpofaei43l/Linkedin.-.Causal.AI.-.An.Introduction.part2.rar
https://rapidgator.net/file/ffe03919cca3831c14e3e5399175a8fe/Linkedin.-.Causal.AI.-.An.Introduction.part1.rar
https://rapidgator.net/file/4abc3b1ff5a601b2458f03ab15b5c6e6/Linkedin.-.Causal.AI.-.An.Introduction.part2.rar
https://turbobit.net/50n50b7vz15s/Linkedin.-.Causal.AI.-.An.Introduction.part1.rar.html
https://turbobit.net/zifk4m6mp201/Linkedin.-.Causal.AI.-.An.Introduction.part2.rar.html
What you'll learn
What Causality is
The relationship between Causation and Association
Why RCT's are the golden standard for Causal Inference
Main components of Pearlian Framework for Causality: Ladder of Causation, Causal Graphs, Do-calculus, Structural Causal Models
Machine Learning & Propensity Score-based Causal Effect Estimators
Causal Discovery (Algorithms)
How to estimate Average Causal Effects using observational data (covering the entire end-to-end process)
Requirements
Basic Probability and Statistics knowledge
Description
In this course, you'll learn the foundational components of Causal Artificial Intelligence (Causal AI). More and more people are starting to realise that correlation-focused models are not enough to answer our most important business questions. Business decision-making is all about understanding the effect different decisions have on outcomes, and choosing the best option. We can't understand the effect decisions have on outcomes with just correlations; we must understand cause and effect. Unfortunately, there is a huge gap of knowledge in causal techniques among people working in the data & statistics industry. This means that causal problems are often approached with correlation-focused models, which results in sub-optimal or even poor solutions. In recent years, the field of Causality has evolved significantly, particularly due to the work of Judea Pearl. Judea Pearl has created a framework that provides clear and general methods we can use to understand causality and estimate causal effects using observational data. Combining his work with advances in AI has given rise to the field of Causal Artificial Intelligence.Causal AI is all about using AI models to estimate causal effects (using observational data). Generally, businesses rely only on experimentation methods like Randomized Controlled Trials (RCTs) and A/B tests to determine causal effects. Causal AI now adds to this by offering tools to estimate causal effects using observational data, which is more commonly available in business settings. This is particularly valuable when experimentation is not feasible or practical, making it a powerful tool for businesses looking to use their existing data for decision-making.This course is designed to bridge the knowledge gap in causal techniques for individuals interested in data and statistics. You will learn the foundational components of Causal AI, with a specific focus on the Pearlian Framework. Key concepts covered include The Ladder of Causation, Causal Graphs, Do-calculus, and Structural Causal Models. Additionally, the course will go into various estimation techniques, incorporating both machine learning and propensity score-based estimators. Last, you'll learn about methods we can use to obtain Causal Graphs, a process called Causal Discovery.By the end of this course, you'll be fully equipped with all tools needed to estimate average causal effects using observational data. We believe that everyone working in the data and statistics field should understand causality and be equipped with causal techniques. By educating yourself early in this area, you will set yourself apart from others in the field. If you have a basic understanding of probability and statistics and are interested in learning about Causal AI, this course is perfect for you!
Overview
Section 1: Causality, Association & RCT's
Lecture 1 Welcome
Lecture 2 Course Slides
Lecture 3 What is Causal AI?
Lecture 4 Simpson's Paradox
Lecture 5 The Need for Causality in Business
Lecture 6 Causation and its relation to Association
Lecture 7 RCT's: The Golden Standard for Causal Inference
Lecture 8 Course Outline
Section 2: The Ladder of Causation
Lecture 9 Introduction
Lecture 10 Layer 1 Explained
Lecture 11 Layer 1 Techniques
Lecture 12 Layer 2 Explained
Lecture 13 Layer 2 Techniques
Lecture 14 Layer 3 Explained
Lecture 15 Layer 3 Techniques
Lecture 16 Do-operator in light of Structural Causal Models
Lecture 17 Recap
Section 3: Causal Directed Acyclic Graphs
Lecture 18 Introduction
Lecture 19 What are Causal DAGs?
Lecture 20 Do-operator in light of Causal DAGs
Lecture 21 Graph Independence & Information Flows
Lecture 22 Graph Patterns
Lecture 23 Blocking Paths & D-separation
Lecture 24 From Graph (In)dependence to Statistical (In)dependence
Lecture 25 Recap
Section 4: Causal Inference Part 1: Identification
Lecture 26 Introduction
Lecture 27 Estimand & Conditional Ignorability
Lecture 28 Probabilities as the foundation of Causal Quantities
Lecture 29 Backdoor Adjustment
Lecture 30 Frontdoor Adjustment
Lecture 31 Do-calculus
Lecture 32 Positivity/Unconfoundedness Trade-Off
Lecture 33 Recap
Section 5: Causal Inference Part 2: Estimation
Lecture 34 Introduction
Lecture 35 Causal Quantities of Interest
Lecture 36 S-Learner
Lecture 37 T-Learner
Lecture 38 X-Learner
Lecture 39 Matching
Lecture 40 Inverse Probability Weighting
Lecture 41 Systematic vs. Random Errors
Lecture 42 Recap
Section 6: Causal Discovery
Lecture 43 Introduction
Lecture 44 Domain Expertise
Lecture 45 Causal Discovery Algorithms: Categories
Lecture 46 Causal Discovery Algorithms: Assumptions
Lecture 47 Constraint-based Causal Discovery
Lecture 48 Score-based Causal Discovery
Lecture 49 Function-based Causal Discovery
Lecture 50 Continuous Optimization-based Causal Discovery
Lecture 51 Causal Discovery in Practice: Hybrid & Iterative
Lecture 52 Recap
Section 7: Closure
Lecture 53 Introduction
Lecture 54 Challenges with Causal AI
Lecture 55 Considerations, Recommendations & Closure
Everyone interested in learning about Causal AI and who has some basic knowledge of Probability and Statistics,Particularly relevant for those working in the Data & Statistics field, like Data Scientists, Data Analysts, Decision Scientists, Statisticians, Data Engineers, Machine Learning Engineers, Computer Scientists, Business Intelligence Analysts, Quantitative Analysts, etc.,Those who want to be at the forefront of advancements in Data and AI for decision-making
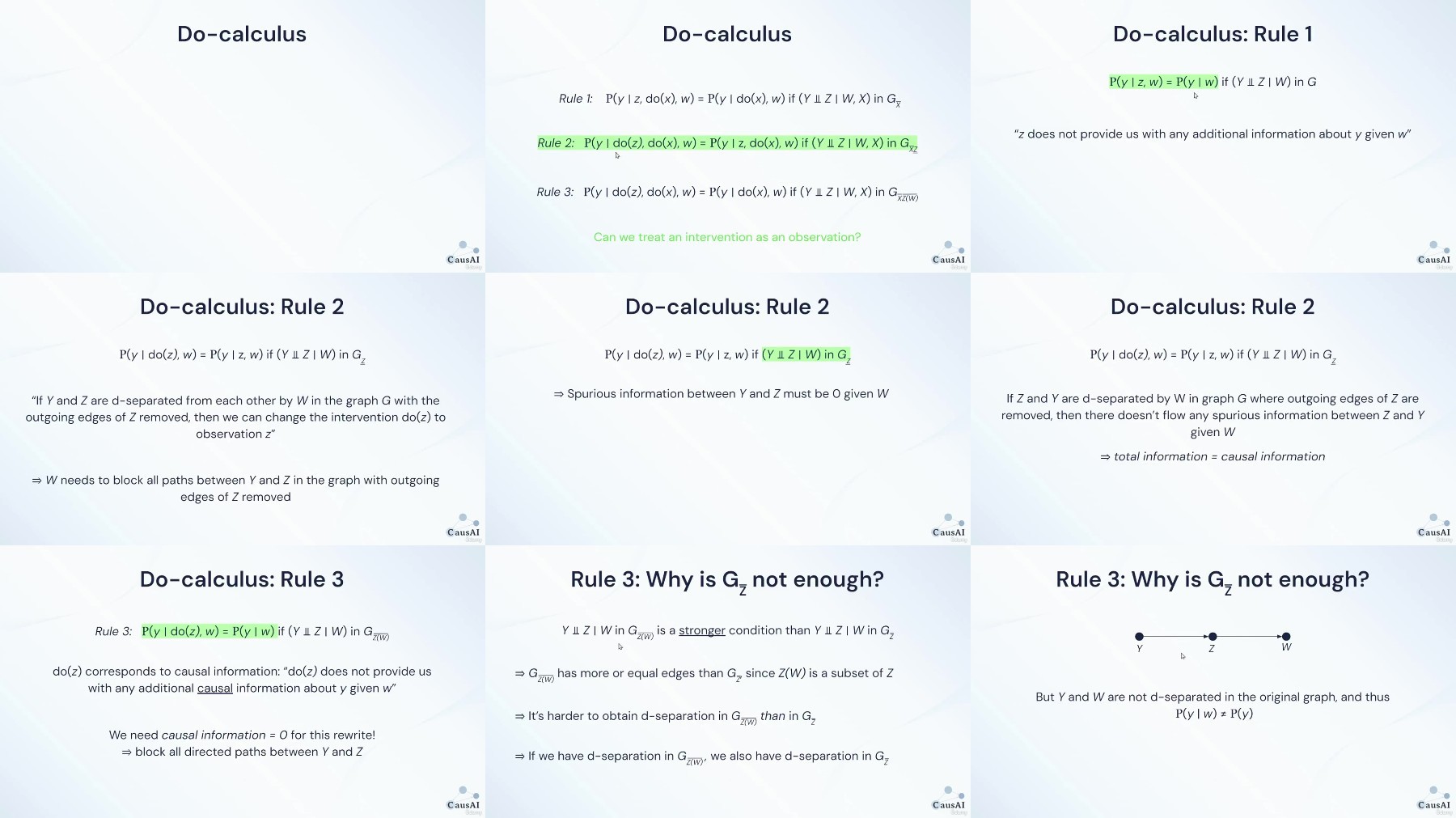
https://ddownload.com/mki84apsb1bo/Linkedin.-.Causal.AI.-.An.Introduction.part1.rar
https://ddownload.com/kulpofaei43l/Linkedin.-.Causal.AI.-.An.Introduction.part2.rar
https://rapidgator.net/file/ffe03919cca3831c14e3e5399175a8fe/Linkedin.-.Causal.AI.-.An.Introduction.part1.rar
https://rapidgator.net/file/4abc3b1ff5a601b2458f03ab15b5c6e6/Linkedin.-.Causal.AI.-.An.Introduction.part2.rar
https://turbobit.net/50n50b7vz15s/Linkedin.-.Causal.AI.-.An.Introduction.part1.rar.html
https://turbobit.net/zifk4m6mp201/Linkedin.-.Causal.AI.-.An.Introduction.part2.rar.html
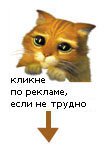