Avoiding Failure In Ai Projects
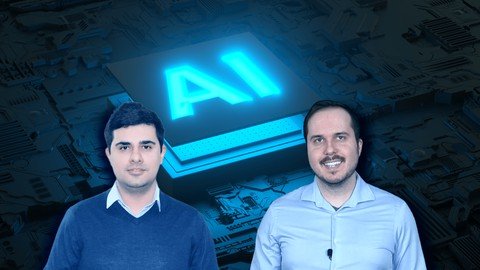
Avoiding Failure In Ai Projects
Published 5/2023
MP4 | Video: h264, 1280x720 | Audio: AAC, 44.1 KHz
Language: English | Size: 1.18 GB | Duration: 1h 20m
Best Practices and Strategies, with Case Studies and Real World Applications
What you'll learn
Strategies on how to kick-off and manage AI projects
Tips to obtain business/process understanding to enhance business engagement
Efficient deployment of AI projects to transform the business
Application of FMEA and ChatGPT to assess project risks and develop mitigation plans
Requirements
This course does not directly teach technical skills like programming, machine learning or data engineering concepts. To get the most knowledge from this course, you must already have a certain level of technical knowledge about your role in a data or AI team. It's recommended to take this course after you are more comfortable with standard prerequisites for data analytics, data management or IT positions.
Description
With the growing recognition of data's value and the potential of machine learning and artificial intelligence, organizations are eager to leverage these technologies. However, a staggering 87% of AI projects fail to make it into production, highlighting the need for a systematic approach to avoid pitfalls and make informed career decisions. This course aims to increase awareness about the most common pitfalls of AI projects and provide a strategic guideline to reduce the risk of failure and enhance user-engagement. The course covers essential strategies for effective AI project management, including project kick-off, obtaining business/process understanding, and efficient deployment of AI solutions. Participants will learn about the importance of clear project goals and deliverables, domain knowledge integration, and communication and collaboration with stakeholders. Practical insights on end-user engagement, quantifying project success, and applying Failure Mode and Effect Analysis (FMEA) and chatGPT for risk assessment and mitigation are also provided.While the course does not directly teach technical skills like programming or machine learning, it is recommended for individuals with a certain level of technical knowledge in data analytics, data management, or IT positions.By the end of the course, participants will have gained valuable knowledge and practical tools to reduce the risk of failure, enhance user engagement, and make informed decisions throughout the project lifecycle. The course equips learners with strategies to transform AI projects into successful endeavors that align with business objectives and deliver tangible benefits. With this newfound expertise, participants can take their projects and teams to the next level in the dynamic landscape of data science.
Overview
Section 1: Introduction
Lecture 1 Course Introduction and Motivation
Section 2: Importance of Clear Project Goals and Deliverables
Lecture 2 Key Failure Modes in AI Projects
Section 3: Strategies for Effective AI-Centered Project Planning and Management
Lecture 3 Lecture Introduction and Overview
Lecture 4 Generic Guideline for Successful AI Project Planning
Section 4: Importance of Domain Knowledge in AI Initiatives
Lecture 5 Lecture Introduction and Overview
Lecture 6 Techniques for Developing Process Understanding
Lecture 7 Case Study of Successful AI Projects that Leveraged Process Understanding
Section 5: Efficiently Deploying AI Products: Scalability, Practicality & Maintainability
Lecture 8 Overview of the Importance of Efficient Deployment
Lecture 9 Practicality Checklist for an AI Product
Lecture 10 Scalability of AI Products: Challenges and Strategies
Lecture 11 Best Practices for Maintenance of AI Product
Section 6: Effective Communication and Collaboration with Stakeholders
Lecture 12 Lecture Introduction and Overview
Lecture 13 Strategies for Effective Communication and Collaboration
Section 7: Transforming Work Processes by End-User Engagement
Lecture 14 Lecture Introduction and Overview
Lecture 15 Strategies to Engage End-Users in AI Projects
Section 8: Quantifying Success in AI Projects: Challenges and Strategies
Lecture 16 Case Study: AI-Based Store Operation Pilot
Section 9: Application of Failure Mode and Effect Analysis (FMEA) in AI Projects
Lecture 17 Lecture Introduction and Overview
Lecture 18 Assessing Severity, Occurrence, and Detectability of Failure Modes
Lecture 19 Case study of FMEA in AI Projects
Section 10: Integration of ChatGPT in Project Workflows
Lecture 20 How ChatGPT can Help with Project Workflows?
Lecture 21 Case Study: Detection Software to Identify COVID-19 Cases
Anyone who wants to know about challenges of AI projects.,Team leads and project managers involved in deployment of digital products,Data Scientists and data engineers who want to to be aware of non-technical challenges to adjust their work,Students who want to learn about real-world challenges of deploying an AI product,IT professionals who support data science projects
HOMEPAGE
https://www.udemy.com/course/avoiding-failure-in-ai-projects/
DOWNLOAD
https://rapidgator.net/file/9375a012b6fb18856033ad51ac889090/Avoiding_Failure_in_AI_Projects.part1.rar.html
https://rapidgator.net/file/d6a0f445dc68282857f9ea7c0bdeb0bc/Avoiding_Failure_in_AI_Projects.part2.rar.html
https://uploadgig.com/file/download/Dc514072cCd145Ac/Avoiding_Failure_in_AI_Projects.part1.rar
https://uploadgig.com/file/download/73c8bAfbEc02dc02/Avoiding_Failure_in_AI_Projects.part2.rar
https://rapidgator.net/file/d6a0f445dc68282857f9ea7c0bdeb0bc/Avoiding_Failure_in_AI_Projects.part2.rar.html
https://uploadgig.com/file/download/Dc514072cCd145Ac/Avoiding_Failure_in_AI_Projects.part1.rar
https://uploadgig.com/file/download/73c8bAfbEc02dc02/Avoiding_Failure_in_AI_Projects.part2.rar
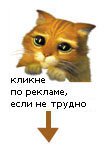